The watershed transform is a computer vision algorithm that serves for image segmentation. This method can extract image objects and separate foreground from background. This tutorial shows how can implement Watershed transformation via Meyer’s flooding algorithm.
How does the Watershed works
The Watershed algorithm is inspired by the representation of geological surfaces, dividing the image into two sets: catchment basins and watershed lines. Our implementation is based on Meyer’s flooding technique, introduced by F. Meyer in the early 1990s.
The algorithm operates on grayscale images. When applied to a gradient image, the basins emerge at the edges of objects. The following steps outline the process:
- Initialization: Individual groups of objects are initialized using pre-selected markers.
- Priority Queue Setup: The adjacent pixels from each group are extracted and added to a priority queue. In classical algorithms, the priority level corresponds to the pixel gradient value, but alternative weight measures can also be employed.
- Processing Pixels: Retrieve and process the lowest priority pixels first from the queue.
- Label Assignment: If all neighboring pixels of the current pixel share the same label, the current pixel is assigned that label.
- Queue Update: Update the priority queue with any unmarked neighboring pixels.
- Iteration: Repeat step 3 until all pixels have been processed.
Finally, all unlabeled pixels describe objects boundaries (the watershed lines).
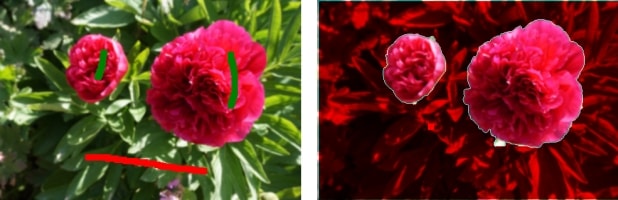
Initialize Segmentation
Initially, the algorithm must select starting points from which to start segmentation. A common way to select markers is the gradient local minimum. However, there are different strategies for choosing seed points. Our HTML5 demo application implements user-controlled markers selection.
Priority Queue Details
The HTML5 realization of Watershed Image Segmentation is based on our custom JavaScript priority queue object (PQueue
). While extracting the pixels, we take the neighbors at each point and push them into our queue.
The queue keeps the pixel in the correct order through a simple binary search. During tracing, the flood process divides the image into background and foreground.
Distance between pixels
Typically, algorithms use a gradient image to measure the distance between pixels. In our sample application we use a different weighting function.
One method to calculate pixel weight is by using improved RGB Euclidean distance [2]. The distance between the center point and the selected neighbor is described by the following JavaScript code segment:
function diffRGB(p1, p2){
const rmean = (data[p1 + 0] + data[p2 + 0]) >>> 1;
const r = (data[p1 + 0] - data[p2 + 0]),
g = (data[p1 + 1] - data[p2 + 1]),
b = (data[p1 + 2] - data[p2 + 2]);
return Math.sqrt((((512+rmean)*r*r)>>8) + 4*g*g + (((767-rmean)*b*b)>>8));
}
Watershed image partition
First, we eliminate image noise using a Gaussian filter with a small sigma value. Then, we initialize the image buffer with appropriate label values corresponding to the input seeds.
for (var i = 0, l = seeds.length; seeds[i]; i++){
var p = seeds[i].pixels;
for (var j = 0, c = p.length; j < c; j++){
buff[p[j]] = (i + 1)*80; // For example 80 and 160 grey values
addPoint(p[j]);
}
}
As a next step, we extract all central pixels from our priority queue until we process the whole image:
while (!queue.empty()){
var el = queue.pop();
addPoint(el);
}
The adjacent pixels are extracted and placed into the PQueue (Priority Queue) for further processing:
function addPoint(p){
for (var i = 0; i < 4; i++){
const pos = p + dirxy[i];
if (buff[pos] === 0){
buff[pos] = buff[p];
buff[pos + 1] = diffRGB(p, pos);
queue.push(pos, comparator);
}
}
}
Related Source code
Image Segmentation Online
Watershed Segmentation is part of our collection of online tools. You can use it to separate and remove background from images.
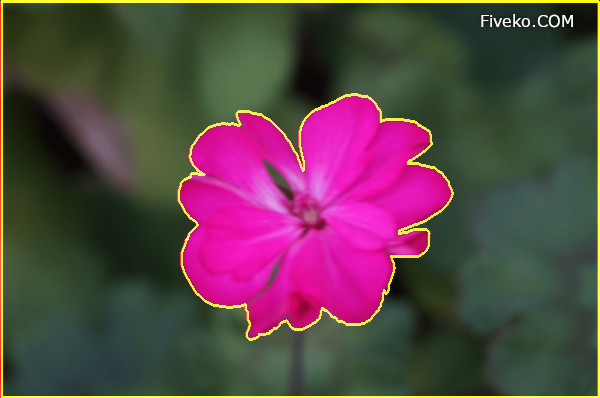
Online Image Tool
See how it works in the browser!